Pandas DataFrame DataFrame.sort_values() Function
-
Syntax of
pandas.DataFrame.sort_values()
: -
Example Codes: Sort DataFrame With Pandas
pandas.DataFrame.sort_values()
Based on a Single Column -
Example Codes: Sort DataFrame With Pandas
DataFrame.sort_values()
Based on Multiple Columns -
Example Codes: Sort DataFrame in Descending Order With Pandas
DataFrame.sort_values()
-
Example Codes: Sort DataFrame by Putting
NaN
First With PandasDataFrame.sort_values()
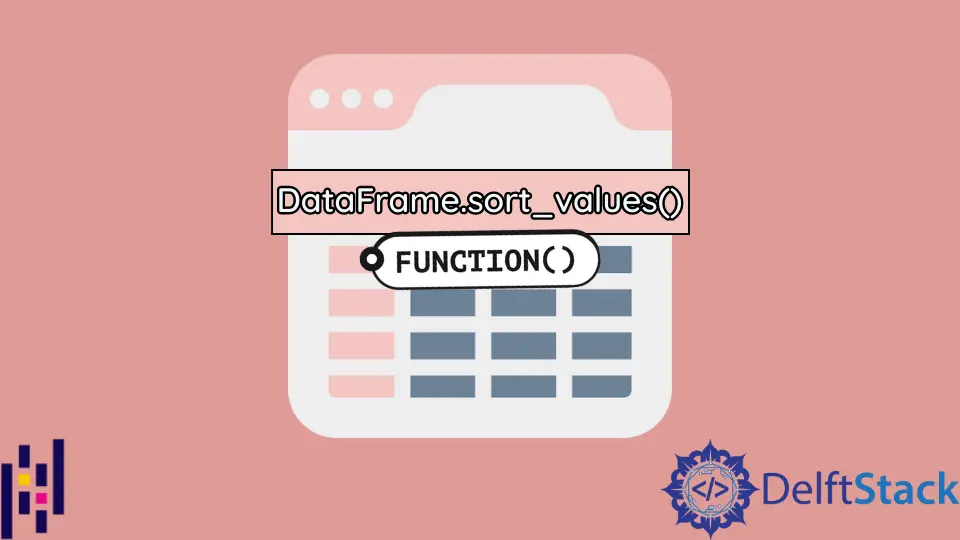
Pandas DataFrame.sort_values()
method sorts the caller DataFrame
in the ascending or descending order by values in the specified column along either index.
Syntax of pandas.DataFrame.sort_values()
:
DataFrame.sort_values(
by,
axis=0,
ascending=True,
inplace=False,
kind="quicksort",
na_position="last",
ignore_index=False,
)
Parameters
by |
Name or list of names to sort by |
axis |
sort along the row (axis=0) or column (axis=1) |
ascending |
sort in ascending order (ascending=True ) or descending order (ascending=False ) |
inplace |
Boolean. If True , modify the caller DataFrame in-place |
kind |
which sorting algorithm to use. default:quicksort |
na_position |
Put NaN value at the beginning (na_position=first ) or the end (na_position=last ) |
ignore_index |
Boolean. If True , the indexes from the original DataFrame is ignored. The default value is False which means the indexes are used. New in version 1.0.0 |
Return
If inplace
is True
, it returns the sorted DataFrame
; otherwise None
.
Example Codes: Sort DataFrame With Pandas pandas.DataFrame.sort_values()
Based on a Single Column
import pandas as pd
dates=['April-10',
'April-11',
'April-12',
'April-13',
'April-14',
'April-16']
sales=[200,300,400,200,300,300]
prices=[3, 1, 2, 4,3,2]
df = pd.DataFrame({'Date':dates ,
'Sales':sales ,
'Price': prices})
print("Before Sorting:")
print(df)
sorted_df=df.sort_values(by=['Price'])
print("After Sorting:")
print(sorted_df)
Output:
Before Sorting:
Date Sales Price
0 April-10 200 3
1 April-11 300 1
2 April-12 400 2
3 April-13 200 4
4 April-14 300 3
5 April-16 300 2
After Sorting:
Date Sales Price
Date Sales Price
1 April-11 300 1
2 April-12 400 2
5 April-16 300 2
0 April-10 200 3
4 April-14 300 3
3 April-13 200 4
It sorts the DataFrame df
in the ascending order (default) by values in the column Price
.
The indexes in the sorted DataFrame
keeps the same as in the original DataFrame
.
If you prefer to have the new index column in the sorted DataFrame
, then you could set ignore_index
(introduced from version 1.0.0) to be True
.
import pandas as pd
dates = ["April-10", "April-11", "April-12", "April-13", "April-14", "April-16"]
sales = [200, 300, 400, 200, 300, 300]
prices = [3, 1, 2, 4, 3, 2]
df = pd.DataFrame({"Date": dates, "Sales": sales, "Price": prices})
print("Before Sorting:")
print(df)
sorted_df = df.sort_values(by=["Price"], ignore_index=True)
print("After Sorting:")
Output:
Before Sorting:
Date Sales Price
0 April-10 200 3
1 April-11 300 1
2 April-12 400 2
3 April-13 200 4
4 April-14 300 3
5 April-16 300 2
After Sorting:
Date Sales Price
0 April-11 300 1
1 April-12 400 2
2 April-16 300 2
3 April-10 200 3
4 April-14 300 3
5 April-13 200 4
Here, we use ignore_index=True
to assign new indexes to rows and ignore the index of the original DataFrame
.
Example Codes: Sort DataFrame With Pandas DataFrame.sort_values()
Based on Multiple Columns
import pandas as pd
dates=['April-10',
'April-11',
'April-12',
'April-13',
'April-14',
'April-16']
sales=[200,300,400,200,300,300]
prices=[3, 1, 2, 4,3,2]
df = pd.DataFrame({'Date':dates ,
'Sales':sales ,
'Price': prices})
print("Before Sorting:")
print(df)
df.sort_values(by=['Sales','Price'],
ignore_index=True,
inplace=True)
print("After Sorting:")
print(df)
Output:
Before Sorting:
Date Sales Price
0 April-10 200 3
1 April-11 300 1
2 April-12 400 2
3 April-13 200 4
4 April-14 300 3
5 April-16 300 2
After Sorting:
Date Sales Price
0 April-10 200 3
1 April-13 200 4
2 April-11 300 1
3 April-16 300 2
4 April-14 300 3
5 April-12 400 2
Here, at first, Sales
is sorted firstly in the ascending order, and then Price
for each Sales
is also sorted in the ascending order.
In the df
, 200
is the smallest value of the Sales
column and 3
is the smallest value of the Price
column for Sales
value of 200
.
So, the row with 200
in the Sales
column and 3
in the Price
goes to the top.
Due to inplace=True
, the original DataFrame
is modified after calling sort_values()
function.
Example Codes: Sort DataFrame in Descending Order With Pandas DataFrame.sort_values()
import pandas as pd
dates=['April-10',
'April-11',
'April-12',
'April-13',
'April-14',
'April-16']
sales=[200,300,400,200,300,300]
prices=[3, 1, 2, 4,3,2]
df = pd.DataFrame({'Date':dates ,
'Sales':sales ,
'Price': prices})
print("Before Sorting:")
print(df)
sorted_df=df.sort_values(by=['Sales'],
ignore_index=True,
ascending=False)
print("After Sorting:")
print(sorted_df)
Output:
Before Sorting:
Date Sales Price
0 April-10 200 3
1 April-11 300 1
2 April-12 400 2
3 April-13 200 4
4 April-14 300 3
5 April-16 300 2
After Sorting:
Date Sales Price
0 April-12 400 2
1 April-11 300 1
2 April-14 300 3
3 April-16 300 2
4 April-10 200 3
5 April-13 200 4
It sorts the DataFrame df
in the descending order of values of column Sales
.
400
is the largest value in the Sales
column; hence the entry goes to the top, and other rows are sorted accordingly.
Example Codes: Sort DataFrame by Putting NaN
First With Pandas DataFrame.sort_values()
import pandas as pd
dates=['April-10',
'April-11',
'April-12',
'April-13',
'April-14',
'April-16']
sales=[200,300,400,200,300,300]
prices=[3, 1, 2, 4,3,2]
df = pd.DataFrame({'Date':dates ,
'Sales':sales ,
'Price': prices})
print("Before Sorting:")
print(df)
sorted_df=df.sort_values(by=['Price'],ignore_index=True,na_position='first')
print("After Sorting:")
print(sorted_df)
Output:
Before Sorting:
Date Sales Price
0 April-10 200 NaN
1 April-11 300 1.0
2 April-12 400 2.0
3 April-13 200 4.0
4 April-14 300 3.0
5 April-16 300 NaN
After Sorting:
Date Sales Price
0 April-10 200 NaN
1 April-16 300 NaN
2 April-11 300 1.0
3 April-12 400 2.0
4 April-14 300 3.0
5 April-13 200 4.0
By default, NaN
values are placed at the end of DataFrame
after sorting.
But by setting na_position=first
, we can place the NaN
values at the beginning of DataFrame
.
Suraj Joshi is a backend software engineer at Matrice.ai.
LinkedIn