Pandas DataFrame DataFrame.append() Function
-
Syntax of
pandas.DataFrame.append()
Method: -
Example Codes: Append Two DataFrames With
pandas.DataFrame.append()
-
Example Codes: Append DataFrames and Ignore the Index With
pandas.DataFrame.append()
-
Set
verify_integrity=True
inDataFrame.append()
Method - Example Codes: Append Dataframe With Different Column(s)
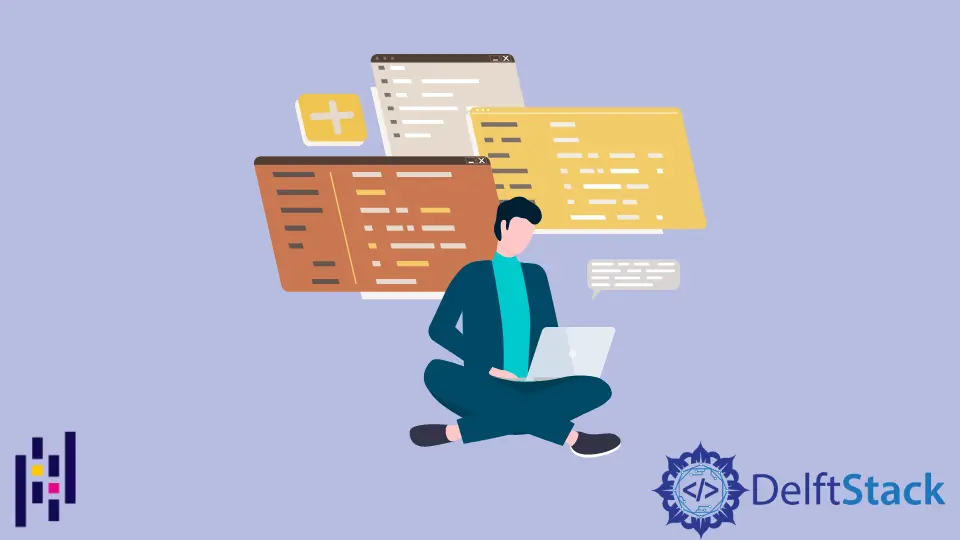
pandas.DataFrame.append()
takes a DataFrame as input and merges its rows with rows of DataFrame calling the method finally returning a new DataFrame. If any column in input DataFrame is not present in caller DataFrame, then the columns are added to DataFrame, and the missing values are set to NaN
.
Syntax of pandas.DataFrame.append()
Method:
DataFrame.append(other, ignore_index=False, verify_integrity=False, sort=False)
Parameters
other |
Input DataFrame or Series, or Python Dictionary-like whose rows are to be appended |
ignore_index |
Boolean. If True , the indexes from the original DataFrame is ignored. The default value is False which means the indexes are used. |
verify_integrity |
Boolean. If True , raise ValueError on creating index with duplicates. The default value is False . |
sort |
Boolean. It sorts the original and the other DataFrame if the columns are not aligned. |
Example Codes: Append Two DataFrames With pandas.DataFrame.append()
import pandas as pd
names_1=['Hisila', 'Brian','Zeppy']
salary_1=[23,30,21]
names_2=['Ram','Shyam',"Hari"]
salary_2=[22,23,31]
df_1 = pd.DataFrame({'Name': names_1, 'Salary': salary_1})
df_2 = pd.DataFrame({'Name': names_2, 'Salary': salary_2})
merged_df = df_1.append(df_2)
print(merged_df)
Output:
Name Salary
0 Hisila 23
1 Brian 30
2 Zeppy 21
Name Salary
0 Ram 22
1 Shyam 23
2 Hari 31
Name Salary
0 Hisila 23
1 Brian 30
2 Zeppy 21
0 Ram 22
1 Shyam 23
2 Hari 31
It appends df_2
at the end of df_1
and returns merged_df
merging rows of both DataFrames. Here, the indices of merged_df
are the same as their parent DataFrames.
Example Codes: Append DataFrames and Ignore the Index With pandas.DataFrame.append()
import pandas as pd
names_1=['Hisila', 'Brian','Zeppy']
salary_1=[23,30,21]
names_2=['Ram','Shyam',"Hari"]
salary_2=[22,23,31]
df_1 = pd.DataFrame({'Name': names_1, 'Salary': salary_1})
df_2 = pd.DataFrame({'Name': names_2, 'Salary': salary_2})
merged_df = df_1.append(df_2,ignore_index=True)
print(df_1)
print(df_2)
print( merged_df)
Output:
Name Salary
0 Hisila 23
1 Brian 30
2 Zeppy 21
Name Salary
0 Ram 22
1 Shyam 23
2 Hari 31
Name Salary
0 Hisila 23
1 Brian 30
2 Zeppy 21
3 Ram 22
4 Shyam 23
5 Hari 31
It appends df_2
at end of df_1
and here the merged_df
gets completely new indices by using ignore_index=True
argument in append()
method.
Set verify_integrity=True
in DataFrame.append()
Method
If we set verify_integrity=True
in append()
method, we get the ValueError
for duplicate indices.
import pandas as pd
names_1=['Hisila', 'Brian','Zeppy']
salary_1=[23,30,21]
names_2=['Ram','Shyam',"Hari"]
salary_2=[22,23,31]
df_1 = pd.DataFrame({'Name': names_1, 'Salary': salary_1})
df_2 = pd.DataFrame({'Name': names_2, 'Salary': salary_2})
merged_df = df_1.append(df_2,verify_integrity=True)
print(df_1)
print(df_2)
print( merged_df)
Output:
ValueError: Indexes have overlapping values: Int64Index([0, 1, 2], dtype='int64')
It generates a ValueError
because the elements in df_1
and df_2
have the same indices by default. To prevent this error, we use the default value of verify_integrity
i.e. verify_integrity=False
.
Example Codes: Append Dataframe With Different Column(s)
If we append a DataFrame
with a different column, this column is added to the resulted DataFrame,
and the corresponding cells of the non-existing columns in the original or the other DataFrame
are set to be NaN
.
import pandas as pd
names_1=['Hisila', 'Brian','Zeppy']
salary_1=[23,30,21]
names_2=['Ram','Shyam',"Hari"]
salary_2=[22,23,31]
Age=[30,31,33]
df_1 = pd.DataFrame({'Name': names_1, 'Salary': salary_1})
df_2 = pd.DataFrame({'Name': names_2, 'Salary': salary_2,"Age":Age})
merged_df = df_1.append(df_2, sort=False)
print(df_1)
print(df_2)
print( merged_df)
Output:
Name Salary
0 Hisila 23
1 Brian 30
2 Zeppy 21
Name Salary Age
0 Ram 22 30
1 Shyam 23 31
2 Hari 31 33
Name Salary Age
0 Hisila 23 NaN
1 Brian 30 NaN
2 Zeppy 21 NaN
0 Ram 22 30.0
1 Shyam 23 31.0
2 Hari 31 33.0
Here, the rows of df_1
get NaN
values for the Age
column because the Age
column is present only in df_2
.
We also set sort=False
to silence the warning that sorting will be deprecated in the future Pandas version.
Suraj Joshi is a backend software engineer at Matrice.ai.
LinkedIn