How to Create a List With a Specific Size in Python
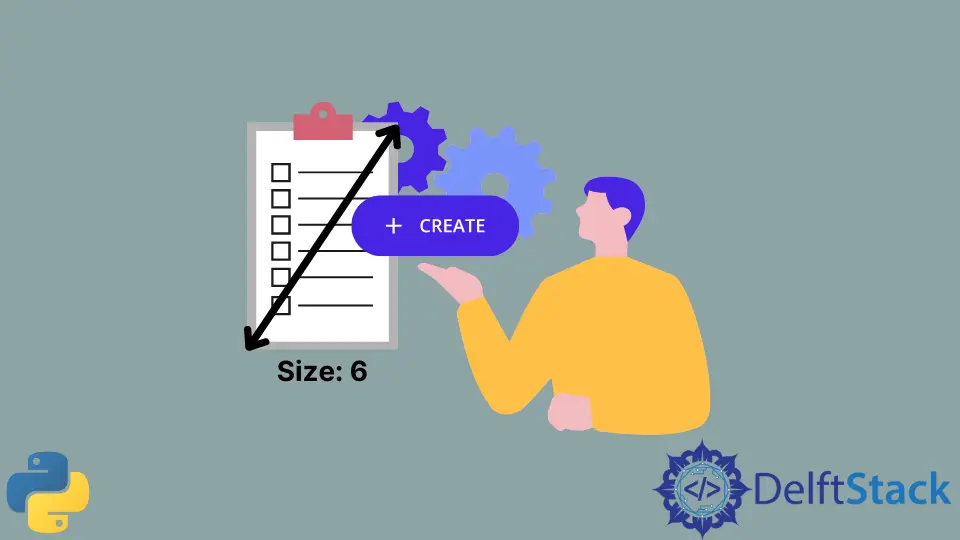
Preallocating storage for lists or arrays is a typical pattern among programmers
when they know the number of elements ahead of time.
Unlike C++ and Java, in Python, you have to initialize all of your pre-allocated storage with some values. Usually, developers use false values for that purpose, such as None
, ''
, False
, and 0
.
Python offers several ways to create a list of a fixed size, each with
different performance characteristics.
To compare performances of different approaches, we will use Python’s standard
module timeit
.
It provides a handy way to measure run times of small chunks of Python code.
Preallocate Storage for Lists
The first and fastest way to use the *
operator, which repeats a list a specified
number of times.
>>> [None] * 10
[None, None, None, None, None, None, None, None, None, None]
A million iterations (default value of iterations in timeit
) take approximately
117 ms.
>>> timeit("[None] * 10")
0.11655918900214601
Another approach is to use the range
built-in function with a list comprehension.
>>> [None for _ in range(10)]
[None, None, None, None, None, None, None, None, None, None]
It’s almost six times slower and takes 612 ms second per million iterations.
>>> timeit("[None for _ in range(10)]")
0.6115895550028654
The third approach is to use a simple for
loop together with the list.append()
.
>>> a = []
>>> for _ in range(10):
... a.append(None)
...
>>> a
[None, None, None, None, None, None, None, None, None, None]
Using loops is the slowest method and takes 842 ms to complete a million iterations.
>>> timeit("for _ in range(10): a.append(None)", setup="a=[]")
0.8420009529945673
Preallocate Storage for Other Sequential Data Structures
Since you’re preallocating storage for a sequential data structure, it may make a lot of sense to use the array built-in data structure instead of a list.
>>> from array import array
>>> array('i',(0,)*10)
array('i', [0, 0, 0, 0, 0, 0, 0, 0, 0, 0])
As we see below, this approach is second fastest after [None] * 10
.
>>> timeit("array('i',(0,)*10)", setup="from array import array")
0.4557597979946877
Let’s compare the above pure Python approaches to the NumPy Python package for scientific computing.
>>> from numpy import empty
>>> empty(10)
array([0., 0., 0., 0., 0., 0., 0., 0., 0., 0.])
The NumPy way takes 589 ms per million iterations.
>>> timeit("empty(10)", setup="from numpy import empty")
0.5890094790011062
However, the NumPy way will be much faster for more massive lists.
>>> timeit("[None]*10000")
16.059584009999526
>>> timeit("empty(10000)", setup="from numpy import empty")
1.1065983309963485
The conclusion is that it’s best to stick to [None] * 10
for small lists, but switch
to NumPy’s empty()
when dealing with more massive sequential data.
Founder of DelftStack.com. Jinku has worked in the robotics and automotive industries for over 8 years. He sharpened his coding skills when he needed to do the automatic testing, data collection from remote servers and report creation from the endurance test. He is from an electrical/electronics engineering background but has expanded his interest to embedded electronics, embedded programming and front-/back-end programming.
LinkedIn FacebookRelated Article - Python List
- How to Convert a Dictionary to a List in Python
- How to Remove All the Occurrences of an Element From a List in Python
- How to Remove Duplicates From List in Python
- How to Get the Average of a List in Python
- What Is the Difference Between List Methods Append and Extend
- How to Convert a List to String in Python