Implémentation de l'algorithme de Viterbi en Python
Vaibhav Vaibhav
4 décembre 2021
Python
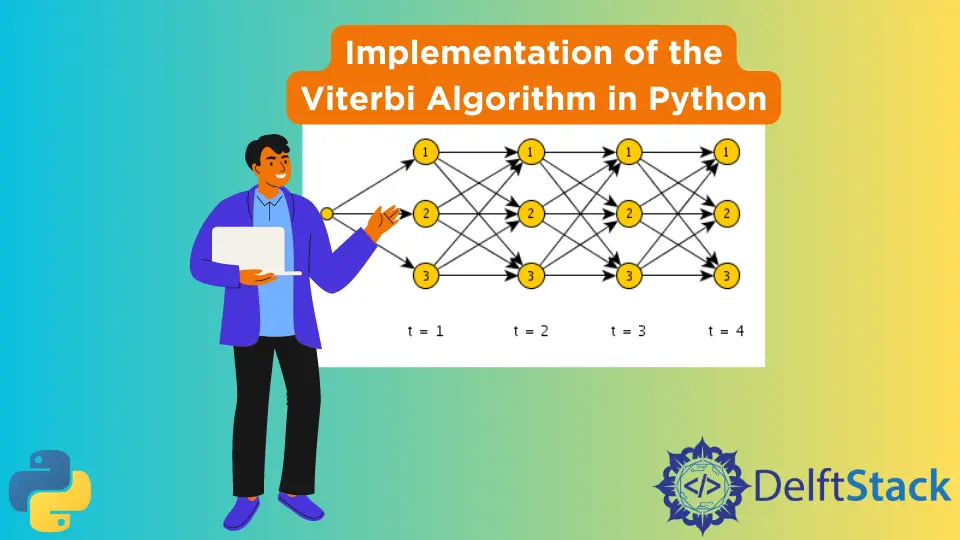
L’algorithme de Viterbi est utilisé pour trouver la séquence d’états la plus probable avec la probabilité a posteriori maximale. C’est un algorithme dynamique basé sur la programmation. Cet article expliquera comment nous pouvons implémenter l’algorithme de Viterbi à l’aide de Python. Nous utiliserons NumPy
pour l’implémentation.
Implémentation Python de l’algorithme de Viterbi
Le code suivant implémente l’algorithme de Viterbi en Python. C’est une fonction qui accepte 4 paramètres qui sont les suivants -
y
: C’est la séquence d’états d’observation.A
: C’est la matrice de transition d’état.B
: C’est la matrice d’émission.initial_probs
: Ce sont les probabilités d’état initial.
Et la fonction renvoie 3 valeurs comme suit -
x
: estimation de probabilité maximale a posteriori de la trajectoire d’état caché, conditionnée à la séquence d’observation y sous les paramètres du modèleA
,B
,initial_probs
.T1
: La probabilité du chemin le plus probable.T2
: La probabilité du chemin le plus probable.
import numpy as np
def viterbi(y, A, B, initial_probs=None):
K = A.shape[0]
initial_probs = initial_probs if initial_probs is not None else np.full(K, 1 / K)
T = len(y)
T1 = np.empty((K, T), "d")
T2 = np.empty((K, T), "B")
T1[:, 0] = initial_probs * B[:, y[0]]
T2[:, 0] = 0
for i in range(1, T):
T1[:, i] = np.max(T1[:, i - 1] * A.T * B[np.newaxis, :, y[i]].T, 1)
T2[:, i] = np.argmax(T1[:, i - 1] * A.T, 1)
x = np.empty(T, "B")
x[-1] = np.argmax(T1[:, T - 1])
for i in reversed(range(1, T)):
x[i - 1] = T2[x[i], i]
return x, T1, T2
Vous aimez nos tutoriels ? Abonnez-vous à DelftStack sur YouTube pour nous aider à créer davantage de tutoriels vidéo de haute qualité. Abonnez-vous
Auteur: Vaibhav Vaibhav